top of page
Machine Learning Assignment Help
Are you looking for Machine Learning Assignment Help OR Coursework Help OR Homework Help? Codersarts machine learning assignment help expert will provides best quality plagiarism free solution at affordable price. We are available 24 * 7 online to assist you. You may chat with us through website chat or email or can fill contact form.
Get Machine Learning assignment help right now and we are ready to deliver your completed assignment within a given time frame.Machine learning programming is quite complicated, and there is nothing wrong or unusual to look for assignment help to deal with it. If you come to Codersarts you will quickly find all the answers you need. Need for help with Machine learning assignment is one of the top priorities of many students at the university.
You can expect a tough time while learning Machine Learning at the beginning. Assignments based on Machine Leaning are quite intensive due to a large number of concepts and you might find yourself in a situation where you need help with Machine Learning assignment. The assignment implementation part is always convoluted, and it keeps students puzzled. That is why codersarts.com has appointed the best programming experts to assist you with Machine learning assignment.Our Machine Learning tutors will ensure that your programming skills improve within a short span.
Key Highlights of assignment solution:
-
Plagiarism Free Solution
-
1:1 Session with Experts
-
Online Tutoring Session
- Code Walkthrough
- Project idea discussion
- Research paper help
Deliverables includes:
-
Source code
-
Screenshots of outputs
-
Short answers to questions
-
Brief report
-
Documentation
-
Detailed report
-
Presentation
Popular services in Machine Learning Assignment Help
Expert Guidance and Custom Solutions from Codersarts
-
Project guidance: Codersarts has experienced mentors who can provide project guidance on Python machine learning projects. Our mentors can help you with project ideation, data preparation, algorithm selection, model training, and evaluation.
-
Project Consultation: Codersarts provides expert consultation on predictive modeling projects, offering advice and recommendations on the best approaches and tools to use.
-
Custom Model Development: We have experienced data scientists and developers who can develop accurate and efficient predictive models, using a range of machine learning algorithms and techniques.
-
Ongoing Support: Codersarts provides ongoing support and maintenance, ensuring that the predictive models are updated and performing optimally over time.
-
Hands-on Projects: We provides hands-on projects that allow individuals to apply what they have learned in a real-world context.
-
Expert Mentorship: We pair individuals with experienced data scientists and developers who serve as mentors, offering guidance and support throughout their training.
-
Deployment and Integration: Codersarts helps businesses integrate the predictive models into their existing systems and processes, and ensures that they are deployed and working optimally.
-
Learning Resources: We provide a wealth of learning resources, including tutorials, articles, and online courses, to help individuals build their knowledge and skills.
-
Offering Custom Solutions: Codersarts can help you create custom solutions for your Python machine learning projects and assignments, tailored to your specific needs and requirements. They can build models from scratch, fine-tune existing models, and integrate machine learning into your existing application.
-
Debugging and Troubleshooting: If you are encountering errors or bugs in your Python machine learning code, Codersarts can help you identify and resolve them. They can help you debug your code, and provide suggestions for improving its performance and accuracy.
What is Machine Learning Assignment Help?
Machine learning assignment is a task of practical learning and Hands-on experience where teacher, professor , instructor assign to students / Machine learning aspirants for assessment as proof of concept. Students face challenges to complete the task as per given instructions and submit on the deadline for evaluations. Machine learning assignment help to map your knowledge with real life use cases of business application.
What is Machine Learning and Why it is important?
Machine learning is a form of AI that enables a system to learn from data rather than through explicit programming. Machine learning uses a variety of algorithms that iteratively learn from data to improve, describe data, and predict outcomes.
Machine learning has now become the regular course of the most college and universities curriculum. Along with web development, mobile app development, Data Structures & Algorithms, and Database skills machine learning are also gaining interested by student and large tech companies because ultimately data and calculation is integral part of business to help in decision making and forecasting and growth, profits and loses.
As a Machine Learning Student or engineer, you can see Machine Learning a technology serving almost every area of technologies. The large volume of data is generated every day by billions of user using the internet. For a human being, it's not possible to process data and get insight from data. These data is very important and profitable for a business to guide customer behavior and activity. Machine learning is a collection of advanced algorithms which process data.
If short, In Machine Learning pipeline we perform following main tasks:
-
Data Collections: Data collection is very step to solve any machine learning assignment and it is the process of gathering and measuring information from countless different sources. In order to use the data we collect to develop practical artificial intelligence (AI) and machine learning solutions, it must be collected and stored in a way that makes sense for the business problem at hand. Most data can be categorized into 4 basic types from a Machine Learning perspective: numerical data, categorical data, time-series data, and text. There are lots of open sources dataset available for machine learning assignment tasks.
-
Data Pre-processing: Data preprocessing in Machine Learning refers to the technique of preparing (cleaning and organizing) the raw data to make it suitable for a building and training Machine Learning models. The steps in data pre processing are data cleaning, data integration, data reduction, and data transformation.
-
Feature Extraction: Feature extraction for machine learning assignment refers to the process of transforming raw data into numerical features that can be processed while preserving the information in the original data set. It yields better results than applying machine learning directly to the raw data. then we do feature selection by choosing a subset of features from the original features so that the feature space is optimally reduced according to a certain criterion. They are used either in isolation or in combination. The sklearn. feature_extraction module can be used to extract features in a format supported by machine learning algorithms from datasets consisting of formats such as text and image.
-
Model Training: A machine learning training model is the phase in the data science development lifecycle where practitioners try to fit the best combination of weights and bias to a machine learning algorithm to minimize a loss function over the prediction range. Training a model simply means learning (determining) good values for all the weights and the bias from labeled examples. In supervised learning, a machine learning algorithm builds a model by examining many examples and attempting to find a model that minimizes loss; this process is called empirical risk minimization.
-
Model Evaluation: Model evaluation is the process of using different evaluation metrics to understand a machine learning model's performance, as well as its strengths and weaknesses. Model evaluation is important to assess the efficacy of a model during initial research phases, and it also plays a role in model monitoring. ROC or Receiver Operating Characteristics Curve is one of the most popular evaluation metrics for checking the performance of a classification model. The curve plots two parameters, True Positive Rate (TPR) and False Positive Rate (FPR).
-
Make Prediction: Prediction mean in Machine Learning refers to the output of an algorithm after it has been trained on a historical dataset and applied to new data when forecasting the likelihood of a particular outcome, such as whether or not a customer will churn in 30 days
The two main challenges of machine learning are data preparation and accurate data collection. Then divide data into labels/Fields or properties. The second task is to select the best model suited for data. To learn and understand machine learning need statistics skills to predict and evaluate data mining results and predictions.
Codersarts machine learning experts are always available and skilled with machine learning pipeline like Data collections, Data pre-processing , features extraction, model training, model evaluation and prediction. If you stuck any of the stage of pipeline we are ready to help you.
We help in following Machine Learning Algorithms as give below:
Have you wondered ever while working on a machine learning assignment or project that Which algorithm is best for machine learning? OR Are machine learning algorithms important to study all of them? OR How will machine learning algorithms improve data quality and results?. Answer is yes and you're not alone at all because selecting the right algorithms are very important steps and that might be subjective to nature of data, industry and biased, hypo thesis and although Comparative studies of many algorithms to get desired result.
Let's take a look of algorithms below
Supervised Learning algorithm
In supervised learning, data is composed of examples where each example has an input element that will be provided to a model and an output or target element that the model is expected to predict. Classification is an example of a supervised learning problem where the target is a label, and regression is an example of a supervised learning problem where the target is a number.
Following are the supervised learning algorithms as given below:
-
Logistic regression Classifier
-
Decision Tree Classifier
-
Random Forest Classifier
-
K nearest neighbor Classifier
-
Support Vector Classifier (SVC)
-
Naive Bayes Classifier
-
AdaBoost Classifier
-
Gradient Boosting Classifier
-
XGB Classifier
-
Linear Regression algorithm
Supervised machine learning algorithms uncover insights, patterns, and relationships from a labeled training dataset – that is, a dataset that already contains a known value for the target variable for each record. Because you provide the machine learning algorithm with the correct answers for a problem during training, the algorithm is able to “learn” how the rest of the features relate to the target, enabling you to uncover insights and make predictions about future outcomes based on historical data.
Writing code for supervised learning algorithms are not always easy tasks sometime you would have to implement from scratch or sometime using machine learning libraries or packages. Getting help in Machine learning project while working on such supervised learning algorithms are time saving and might be worth it. Codersarts machine learning project assistance will help you get it done timely and easily.
Unsupervised Learning algorithm
Unsupervised learning is a type of machine learning in which models are trained using an unlabeled dataset and are allowed to act on that data without any supervision. In unsupervised learning not use the target variable. Unsupervised learning cannot be directly applied to a regression or classification problem because unlike supervised learning, we have the input data but no corresponding output data. The goal of unsupervised learning is to find the underlying structure of the dataset, group that data according to similarities, and represent that dataset in a compressed format.
Types of Un Supervised learning
-
Clustering
-
Association
Clustering
It is a method of grouping the objects into clusters based on the object with most similarities that remains in a group and has less or no similarities with the object of the other group. clustering analysis finds the commonalities between the data objects and categorizes them as per the presence and absence of those commonalities. Clustering algorithms are very important to unsupervised learning and are key elements of machine learning in general. These algorithms give meaning to data that are not labelled and help find structure in chaos. Courses on machine learning will usually start with clustering, because it is both simple and useful. Get a chance to work with machine learning expert and guidance.
Association
An association rule is an unsupervised learning method which is used for finding the relationships between variables in the large database. It determines the set of items that occur together in the dataset. Association rule makes marketing strategy more effective.
-
K-Means Clustering
-
Hierarchical Clustering
-
Principal component analysis
-
Singular value decomposition
-
Independent component analysis
-
Anomaly detection
-
Neural network
-
Apriori algorithm
-
Singular value decomposition (SVD)
Reinforcement Learning algorithm
Reinforcement Learning is a reward based Machine learning technique in which an agent learns to behave in an environment by performing the actions and seeing the results of actions. For each good action, the agent gets positive reward, and for each bad action, the agent gets negative reward or penalty. In Reinforcement Learning, the agent learns automatically using reward without any labeled data.
Reinforcement learning is a type of machine learning method where an intelligent agent (computer program) interacts with the environment and learns to act within that.
Are you working on Reinforcement Learning algorithm assignment in machine learning and need someone to help you clear your doubts when you need most? you can book 1:1 live session with machine learning engineers, experts and researchers or even though can opt the machine learning mentorship program at affordable price.
Semi-Supervised Learning algorithm
This type of algorithm is neither fully supervised nor fully unsupervised. This type of algorithm uses a small supervised learning component i.e small amount of pre-labeled annotated data and large unsupervised learning component i.e. lots of unlabeled data for training.
A Semi-Supervised algorithm assumes the following about the data –
-
Continuity assumption : The algorithm assumes that the points which are closer to each other are more likely to have the same output label.
-
Cluster assumption : The data can be divided into discrete clusters and points in the same cluster are more likely to share an output label.
-
Manifold assumption : the data lie approximately on a manifold of much lower dimension than the input space. This assumption allows the use of distances and densities which are defined on manifolds.
Gradient Boosting algorithms
Gradient boosting algorithms are supervised machine learning techniques for classification and regression problems. It is one of the most powerful algorithms for building predictive analysis.
Gradient boosting involves three elements.
-
A loss function to be optimized.
-
A weak learner to make predictions.
-
An additive model to add weak learners to minimize the loss function.
Many models are trained sequentially. Each new model gradually minimizes the loss function of the whole system using Gradient Descent method. The main idea behind this algorithm is to construct new base learners which can be optimally correlated with the negative gradient of the loss function, relevant to the whole ensemble.
-
Gradient Boosting Machine (GBM)
-
Extreme Gradient Boosting Machine (XGBM)
-
LightGBM
-
CatBoost
Gradient boosting is a greedy algorithm and can overfit a training dataset quickly. It can benefit from regularization methods that penalize various parts of the algorithm and generally improve the performance of the algorithm by reducing overfitting. Gradient Boosting Algorithm is generally used when we want to decrease the Bias error and can be used in regression as well as classification problems. In regression problems, the cost function is MSE whereas, in classification problems, the cost function is Log-Loss.
Are you wondering about what's happening in Gradient boosting algorithm? We've data scientist and machine learning engineers to explain the step by steps code walkthrough. if you would like to see any help as part of machine learning assignment or coursework, just email us at contact@codersarts.com with your complete requirement and we'll do our best to provide Gradient boosting algorithms Expert Help.
Dimensionality Reduction Algorithms.
Dimensionality reduction is an unsupervised learning technique. In machine learning classification problems, there are often too many factors on the basis of which the final classification is done. These factors are basically variables called features. The higher the number of features, the harder it gets to visualize the training set and then work on it. Sometimes, most of these features are correlated, and hence redundant. This is where dimensionality reduction algorithms come into play. Dimensionality reduction is the process of reducing the number of random variables under consideration, by obtaining a set of principal variables. It can be divided into feature selection and feature extraction.
Examples:
-
Principal Component Analysis
-
Singular Value Decomposition
-
Linear Discriminant Analysis
-
Isomap Embedding
-
Locally Linear Embedding
-
Modified Locally Linear Embedding
Pretrained model / Transfer Learning
A pre-trained model is a model created by someone else to solve a similar problem. Instead of building a model from scratch to solve a similar problem, you use the model trained on other problems as a starting point. Using the pretrained model techniques is called transfer learning. It has a special feature train for one task and we can use the knowledge for another related task.
Some pretrained models' names.
-
VGG16
-
MobileNetV2
-
InceptionResNetV2
-
InceptionV3
-
ResNet50.
Linear Regression
It is a method of modeling the relationship between dependent variable y and independent variable X. Variable may be one or more. When there is only one independent/explanatory variable, it is called Simple Linear Regression and for more variables, it is called Multiple Linear Regression. Linear regression is known for being a simple algorithm and a good baseline to compare more complex models to.
Do you need help in implementation of Linear Regression algorithms or want to learn Linear regression? Codersarts machine learning expert will be available to help you on-demands
Logistic Regression
It is used to determine discrete values like binary value, yes/no or false/true, based on a set of independent variables.
Here some examples are:
-
Spam detection: predict if mail is spam or not
-
Credit card fraud: predict if credit card transaction is fraud or not
-
Marketing: if the user will buy a product or not
Decision Trees
It is very famous and widely used supervised learning algorithm. Decision Tree is a type of Supervised Machine Learning (that is you explain what the input is and what the corresponding output is in the training data) where the data is continuously split according to a certain parameter. The tree can be explained by two entities, namely decision nodes and leaves. Are you looking for Decision trees Expert Help? please share your complete requirement details via email or website chat and our expert will review and let you know price quote?
Support Vector Machine(SVM)
It is used for the classification method. In this method, each plot is placed in n-dimensional space. Here n represents the number of features.
Naive Bayes (NB)
It is also be used for the classification method. This classification method assumes that the features in this method are independent. This classifier assumes that the presence of any particular feature in this class is unrelated to presence of any other given feature.
KNN (k-Nearest Neighbors)
It supports both, classification and a regression method. But it large support for classification problems. It finds the distance from one given instance variable points. It works in the following way:
Loads the data, initializes the value of k. And follow given below procedure
-
Find the distance from instance data and rows in training data.
-
Sorts the calculated distance in ascending order.
-
Gets top k rows from the sorted array.
-
Gets the most frequent class of these rows.
-
Returns predicted class.
k-means
It supports the unsupervised learning algorithm, which is used for unlabelled data. K-means is the simple and easy way to classify a given data set through a number of clusters where k is a number of assumed clusters. How k-means works:
-
Find k-number of points from each cluster, here cluster is work as a centroid.
-
Each data point forms a cluster with the closest centroid.
-
After this find the centroid of each cluster based on members in that cluster. Repeats this step to find new centroids.
-
Finds the nearest distance for each data point from new centroids. Associates it with new k-clusters.
And other Algorithms likes
-
Random Forest
-
Dimensionality Reduction
All these advantages make and remain one of the most popular AI technologies to handle statistical algorithms or powerful usable data predictions in industries. In the internet domain, java’s popularity has increased tremendously, especially on the server-side of the internet. Machine Learning landed top among the most-used in artificial intelligence.
Get help & support from a k-means expert in machine learning assignment and k-means algorithms implementations from scratch.
Feature extraction Techniques in Machine Learning
Feature extraction involves reducing the number of resources required to describe a large set of data.
When the input data to an algorithm is too large to be processed and it is suspected to be redundant
then it can be transformed into a reduced set of features (also named a feature vector). Determining a subset of the initial features is called feature selection/extraction.The selected features are expected to contain the relevant information from the input data, so that the desired task can be performed by using this reduced representation instead of the complete initial data.
-
Bag of words
-
Auto-encoders
-
Countvectorizer
-
TfIdf Vectorizer
-
Hashing Vectorizer
-
Kernel PCA
-
Partial least squares
-
Semidefinite embedding
-
Latent semantic analysis (LSA)
-
t-distributed Stochastic Neighbor Embedding (t-SNE)
-
Multilinear subspace learning
-
Nonlinear dimensionality reduction
-
Multifactor Dimensionality reduction
-
Locally Linear Embedding (LLE)
-
Linear Discriminant Analysis (LDA)
-
Principal Component Analysis (PCA)
-
Multilinear Principal Component Analysis
-
Independent Component Analysis (ICA)
Most frequently used libraries in Machine Learning Assignment
Sklearn
Scikit-learn is a free software machine learning library for the Python programming language. It features various classification, regression and clustering algorithms including support vector machines, random forest, gradient boosting, k-means and DBSCAN, and is designed to interoperate with the Python numerical and scientific libraries NumPy and SciPy.

SciPy
SciPy is a free and open-source Python library used for scientific computing and technical computing. SciPy contains modules for optimization, linear algebra, integration, interpolation, special functions, FFT, signal and image processing, ODE solvers and other tasks common in science and engineering.

NumPy
NumPy is a library for the Python programming language, adding support for large, multi-dimensional arrays and matrices, along with a large collection of high-level mathematical functions to operate on these arrays.

Matplotlib
Matplotlib is a plotting library for the Python programming language and its numerical mathematics extension NumPy. It provides an object-oriented API for embedding plots into applications using general-purpose GUI toolkits like Tkinter, wxPython, Qt, or GTK

Seaborn
Seaborn is a library mostly used for statistical plotting in python. It is built on top of matplotlib.

AWS Machine Learning Assignment Help
Are your Machine Learning assignment using AWS machine learning services and you need help? Are you looking to solve a Machine Learning assignment using AWS? Do you want to learn AWS for Machine learning project deployment? Or do you want to customize your machine learning journey? We have AWS machine learning expert to help find one that interests you.
Make accurate predictions, get deeper insights from your data, reduce operational overhead, and model deployment. Codersarts helps you at every stage of your ML adoption journey with the most comprehensive set of artificial intelligence (AI) and ML services, infrastructure, and implementation resources.
some basic task in AWS for Machine learning assignment is create notebook instance, select the right kernel , train & test and finally creating endpoint. Such one service is Amazon SageMaker ML service enabling data scientists, data engineers, MLOps engineers, and business analysts to build, train, and deploy ML models for any use case, regardless of ML expertise.
Get live 1:1 session with Machine learning AWS experts—for you or your team
Do you want live training with an AWS expert where you’ll get the chance to ask questions and receive real-time feedback? Do you want the option to schedule training for your team, business, or group? We recommend our Codersarts AWS Training. These are interactive, immersive classes led by expert AWS instructors who provide guided help to individuals and groups, in person or virtually. Discuss your real-world challenges with our instructors in the classroom to reinforce your learning and help you understand how to apply best practices to overcome your challenges.
Google Colab Machine Learning Assignment Help
Colab, or ‘Colaboratory’, allows you to write and execute Python in your browser, with
-
Zero configuration required
-
Free access to GPUs
-
Easy sharing
Whether you're a student, a data scientist or an AI researcher, Colab can make your work easier and an interactive environment called a Colab notebook that lets you write and execute code. With Colab you can harness the full power of popular Python libraries to analyse and visualise data. The code cell below uses numpy to generate some random data, and uses matplotlib to visualise it.
Machine learning with Colab you can import an image dataset, train an image classifier on it, and evaluate the model, all in just a few lines of code. Colab notebooks execute code on Google's cloud servers, meaning you can leverage the power of Google hardware, including GPUs and TPUs, regardless of the power of your machine. All you need is a browser.
Colab is used extensively in the machine learning community with applications including:
-
Getting started with TensorFlow
-
Developing and training neural networks
-
Experimenting with TPUs
-
Disseminating AI research
-
Creating tutorials
Codersarts ML experts are available to help you in the any of above requirement in Google Colab Machine Learning Assignment Help
Machine Learning assignment help using Azure ML Studio
Azure machine learning studio is the service provided by Microsoft Azure to create various machine learning models for your data. Here you do not need to write any code to build a machine learning model. Azure machine learning studio is a drag and drop tool that can be used to publish the machine learning model like web services. This studio provides you interactive and visual workspace where you can drag and drop the dataset and perform the data analysis, and the analysis converted into interactive canvas.
Microsoft Azure provides the services as cloud computing to building, testing, deploying and managing the application. All the facilities are provided by microsoft managed data centres. Read more
If you need implementation for any machine learning assignment using Azure ML Studio. feel free contact us via email, live chat, contact form
Machine Learning Research Assignment Help
Codersarts research team allows you to pursue your interests, while enabling you to implement a new research Idea as they do the heavy lifting and make it easy for you to focus on new ideas and new ways and Gain academic credentials that will help you publish your work in designated journals. To elaborate on what pursuing serious research actually is, we can go through different components of a research paper which indefinitely summarises all the research work
We offers code implementation in Machine learning, developing new methods
At Codersarts, We have a team of Machine learning experts, developers and engineers to help you in the Research Paper Implementation journey at a point of time when you feel the need for either help or it's a 1:1 mentorship or complete solution of the paper with well commented code and documentations. Also you can take help from our experts in presentation or report write up at an affordable price.
Browser more ML Research help
How should student solve Machine Learning Projects Assignment
Aspiring machine learning students want to learn on machine learning projects but struggle hard to find interesting ideas and how to build the project.
How should students build Machine learning projects:
-
Find data science or machine learning project ideas that motivate you
-
When deciding on a machine project to get started.
-
You can decide the domain and dataset based on your interest.
-
Size of the dataset and complexity of the dataset.
-
If you are a fresher or a beginner, We recommend you get started with ML projects that focus on data cleaning and then move on to analytics, machine learning, and deep learning.
Nowadays machine learning is more popular because computation is abundant and cheap. More professionals are pursuing careers in machine learning. One of the best ways to build a career in machine learning is developing a project. For that We have created a list of innovative and interesting machine learning projects and explained it in detail in the videos for those students, who are passionate about machine learning and want to learn.
If you are passionate about machine learning and want to know how to build machine learning projects, you must watch our videos. In this article we have shared detailed information about each project and link of the videos.
Credit Risk Prediction Project Assignment Help Solution
The solution of this project consists of two parts: part 1 and part 2. Let's see below details
Credit Risk Prediction Project Part 1: This is the introduction part of the CREDIT RISK PREDICTION Project where we provide the details and procedures of the coming project that we will build in Part2 of this Project. This is based on prediction of defaulters in bank credit based on the data provided by the bank using past analysis. The result of this project will be that we will be able to forecast what are the chances of a person with certain credentials that will be a defaulter or a successful player.
Credit Risk Prediction Project Part 2: This is the second part of the CREDIT RISK PREDICTION Project where we create a complete project on Kaggle Community Platform regarding prediction of Credit Failure of customers based on their credentials. We use data cleaning, data plotting and utilised Random Forest Classifier, Support Vector Machine and Logistic Regression with best parameters possible for getting the best prediction accuracy. All these algorithms are mathematical implementations and we have utilised them with optimal parameters.
BIKE DEMAND ANALYSIS Project Assignment Help Solution
The solution of this project consists of two parts: part 1 and part 2. Let's see below details
BIKE DEMAND ANALYSIS Project Part 1:This is the introduction part of the BIKE DEMAND ANALYSIS Project where we provide the details and procedures of the coming project that we will build in Part2 of this Series. This is based on analysis of hourly and daily bike demand in a city as Rented Bike Count where we have divided this count into categories and used them to analyse whether for some given conditions like weather, Holiday, Events and seasons, on a particular day or hour what will be the predicted demand of rented bikes. The result would make us more predictable towards what days showcase higher , lower or moderate demand for bikes.
BIKE DEMAND ANALYSIS Project Part 2: This is the second part of the BIKE DEMAND ANALYSIS Project where we create a complete project on Kaggle Community Platform regarding prediction of hourly or daily bike demand based on data over a year for a city. We use data cleaning, data plotting and utilised Random Forest Classifier, Support Vector Machine and Logistic Regression with best parameters possible for getting the best prediction accuracy. All these algorithms are mathematical implementations and we have utilised them with optimal parameters.
PREDICTING WINE QUALITY Project Assignment Help Solution
The solution of this project consists of two parts: part 1 and part 2. Let's see below details
PREDICTING WINE QUALITY Project Part 1:This is the introduction part of PREDICTING WINE QUALITY Project where we provide the details and procedures of the coming project that we will build in Part2 of this Series. This is based on analysis of wine quality based on content or ingredients of the red wine provided such as sulphides, chlorides, alcohol, pH, citric acid etc. and categorise whether the wine is commercially good or not in terms of quality. The result will be that we will be able to analyse the quality of wine with all sets of various values using AI and know whether or not the product is high in quality.
PREDICTING WINE QUALITY Project Part 2: This is the second part of the PREDICTING WINE QUALITY Project where we create a complete project on Kaggle Community Platform regarding prediction of Quality of red wine based on various materials used to create the product. We use data cleaning, data plotting and utilised Random Forest Classifier, Support Vector Machine and Logistic Regression with best parameters possible for getting the best prediction accuracy. All these algorithms are mathematical implementations and we have utilised them with optimal parameters.
HEART ATTACK PREDICTION Project Assignment Help Solution
The solution of this project consists of two parts: part 1 and part 2. Let's see below details
HEART ATTACK PREDICTION Project Part 1 :This is the introduction part of HEART ATTACK PREDICTION Project where we provide the details and procedures of the coming project that we will build in Part2 of this Series. This is based on analysis of a patient’s diagnosis conditions like blood pressure, random sugar, cholesterol, resting pressure, pulse etc. to analyse whether or not the patient is probable for a heart attack risk. The result will be that we will be able to analyse on the basis of a patient's test results whether they have a risk of heart attack or not.
HEART ATTACK PREDICTION Project Part 2: This is the second part of the HEART ATTACK PREDICTION Project where we create complete project on Kaggle Community Platform regarding prediction of Heart attack risk of a patient based on their various test results. We use data cleaning, data plotting and utilised Random Forest Classifier, Support Vector Machine , K Nearest Neighbours and Logistic Regression with best parameters possible for getting the best prediction accuracy. All these algorithms are mathematical implementations and we have utilised them with optimal parameters.
BRAIN TUMOUR DETECTION Project Assignment Help Solution
The solution of this project consists of two parts: part 1 and part 2. Let's see below details
BRAIN TUMOUR DETECTION Project Part 1 :This is the introduction part of BRAIN TUMOUR DETECTION Project where we provide the details and procedures of the coming project that we will build in Part2 of this Series. This is based on classification of an image to know if the MRI image shows any signs of brain tumour or not and if yes, what kind of tumour. The result will be able to help the doctors to analyse the mri images to know the tumour state in the brain.
BRAIN TUMOUR DETECTION Project Part 2: This is the second part of the BRAIN TUMOUR DETECTION Project where we create a complete project on Kaggle Community Platform regarding classification of MRI images into types of Brain tumour or no tumour based on training data. We use Data directory flow, Convolutional Neural Network from Keras and Sequential Model along with OpenCV for creation of our model. As a result we will be able to predict accurately whether the MRI image shows any tumour or not.
For more projects - check it out here
As mentioned above videos we have explained assignment solution step by step. so, there are several ways to get help with machine learning assignments like only solution with code implementation and also can book 1:1 live sessions with our machine learning experts.
How can we assist you today? If you are inquiring about a specific service, please let us know and we will be happy to provide you with more information. If you would like to speak with a representative directly, please provide your contact information and the best time for us to reach you. Also share your machine learning project or assignment details at email id - contact@codersarts.com
We appreciate your business and look forward to helping you.
What type of machine learning assignment or project help looking for?
We Help in these Machine Learning Assignment topics
-
Data Science Assignment Help in Python
-
Pandas & NumPy Assignment Help
-
Exploratory Data Visualization Assignment Help
-
Data Cleaning and Analysis
-
Text Processing in the Command Line
-
APIs & Web Scraping
-
Data Visualization in Python
-
Matplotlib Assignment Help
-
Processing Large Datasets in Pandas
-
Programming Concepts with Python
-
Spark & Map-Reduce
-
Natural Language Processing
-
Kaggle Fundamentals
-
Machine Learning Project
-
Deep Learning: Fundamentals
Dedicated Machine Learning Expert For Assignment Help
At Codersarts.com we offer solutions to all aspect of Machine Learning, Assign ML coders to help you with all your ML needs. You can avail by hiring ML developers and programmers. We provide programming help, coding help, and other ML related algorithms. ML is one of the parts of AI serving the industry from a long time and known for fast areas of availability worldwide. It is the most demanding skill and choice for a developer for many good reasons, and the trend is expected to continue for many years to come and digitization, the need of ML a developer is also increasing day by day.
Basic Machine Learning Assignment Help
In this we provide guidance in following machine learning topics.
-
Regression Techniques
-
Numerical Optimization
-
Introduction to Neural Networks
Feature Extraction Help in Machine Learning
In Machine learning assignment Features extraction and dimensions have their importance and application.
-
Features and Importance
-
Feature scaling
-
The Curse of Dimensionality
-
SVD and Principal Component Analysis
Machine Learning Homework Help
Machine Learning Homework will cover with basic understanding of logic, discussing about Flowcharts, Psudo Code and students will solve also some of the Puzzles in initial classes. Machine Learning Homework expert will answers all the questions and doubts with step-by-step explanations. Our online Machine Learning Homework help tutors are available.
Machine Learning Coursework Help
Machine Learning CourseWork will cover with basic understanding machine learning assignment, teach machine learning online, advanced machine learning assignments, machine learning projects help
Our online Machine Learning Coursework help experts are available.
What is Deep Learning
Deep learning allows machines to solve complex problems even when using a data set that is very diverse, unstructured and inter-connected. The more deep learning algorithms learn, the better they perform.

Deep Learning Expert Help
Deep learning is an important part of machine learning. There are a lots of possibilities of machines learning to do things humans currently do in our factories, warehouses, offices and homes. While the technology is evolving—quickly—along with fears and excitement, terms such as artificial intelligence, machine learning and deep learning may leave you perplexed.
Here are list of common fields applied:
-
Computer vision, speech recognition
-
Natural language processing, audio recognition
-
Social network filtering, machine translation
-
Bioinformatics, drug design, medical image analysis
-
Material inspection and board game programs
where they have produced results comparable to and in some cases superior to human experts.
Mathematical Concepts behind machine learning algorithms
ML Assignment will cover with basic understanding of logic, discussing about Flowcharts, Psudo Code and students will solve also some of the Puzzles in initial classes.
-
Introduction to Machine Learning
-
Python 3.5 overview
-
Linear Algebra
-
Statistics and Probability
-
Numpy, Scipy, and Scientific computation with Python
Algorithms and Data Handling
Our expert are also good at some advanced algorithms such as K-means clustering and Random Forest Classifiers. which is common and taught in most of assignment lectures.
-
Nearest Neighbour search and K-means clustering.
-
Decision trees and Naive Bayes.
-
Data Scraping, Handling, Cleaning
-
Random Forest Classifiers.
Deep Learning concepts
More complex Machine Learning topics and algorithms which help you in solving and optimising solutions of lots of real world problems.
-
Neural Architectures and Training
-
Deep learning methods
-
Convolutions and the GoogLe Net
-
Dimensions revisited: The Auto-encoder
-
Recurrent and Combined Architectures
-
Support Vector Machines
-
Introduction to Unsupervised and Reinforcement Learning
-
Transfer Learning
Machine Learning Projects
Expert will guide to students for building several projects that use the ML techniques to solve a real life problem.
-
Handwritten digit classification
-
Face Recognition
-
Image classification and Object detection
-
Automated music generation
-
Text/Poem generating bot
-
Recommender systems
-
Emotion/Sentiment Analysis
What is TensorFlow
TensorFlow makes it easy for beginners and experts to create machine learning models for desktop, mobile, web, and cloud. See the sections below to get started.
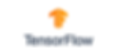
TensorFlow Expert Help
Are you a python developer and want to use TensorFlow library in order to do deep-learning. Or As a company you are looking for TensorFlow Expert Help or Want to Hire TensorFlow Developer. Codersarts is a top rated website for online TensorFlow Assignment Help, Homework help, Coursework Help, coding help in TensorFlow.
Get your project or assignment completed by TensorFlow expert and experienced developers.We have dedicated team of TensorFlow experts to help you create your next machine learning project.
What is Keras
Keras is a high-level neural networks API, written in Python and capable of running on top of TensorFlow, CNTK, or Theano. It was developed with a focus on enabling fast experimentation. Being able to go from idea to result with the least possible delay is key to doing good research.

Keras Expert Help
There are countless deep learning frameworks available today. Why use Keras rather than any other? Here are some of the areas in which Keras compares favorably to existing alternatives.
Codersarts is a top rated website for online Keras Assignment Help, Homework help, Coursework Help, coding help in Keras. Get your project or assignment completed by Keras expert and experienced developers.
Keras prioritizes developer experience:
-
It offers consistent & simple APIs.
-
Keras integrates with lower-level deep learning languages (in particular TensorFlow)
We have dedicated team of Keras experts to help you create your next machine learning project.
Basic skill sets expected when you hire Machine Learning expert:
-
Knowledge of OOPs: Great Machine learning developers should be good in the implementation of object-oriented design patterns.
-
Knowledge of the Core Python: Before start machine learning first necessary need to basic module, control flow and exception, import, and creating packages.
-
Knowledge of the basic algorithm: Have a some knowledge of ML algorithm before start ML.
-
Knowledge of Data Science: You have some knowledges of data science and its libraries.
-
Basic knowledge of statistical data: In machine learning, statistical calculation use operate any data it is necessary to have some basic knowledge of statistics.
Machine Learning important libraries and tools used in assignment:
Depending on the type of tasks, we use different stacks of technology. They may include the following libraries and frameworks:
-
Machine Learning Support technologies: NLP, OpenCV, Artificial neural networks, Support vector machines
-
Machine Learning tools: Setup-tools, pip, etc
-
Test frameworks: UnitTest, py.test, etc
-
Version: Python 3.x
-
Data analysis tools: NumPy, SciPy, SciPy, Matplotlib, Pandas, Scikit-learn (sklearn)
Let's see how Machine Learning is still relevant:
Machine Learning is solutions for all whether you are student, small or Medium level enterprises. You can see Machine Learning everywhere, Machine Learning development happen in the finance and insurance domain, industries, and healthcare.
Here are some of the common usage of Machine Learning in the real world:
-
Image recognition
-
Speech recognition
-
Recommendation systems
-
Medical Diagnosis
-
Statistical Calculations
-
Classification
-
Prediction
-
Extraction
-
Regression
-
Robotics - ROS
Why choose Codersarts machine learning assignment help services
We are a leading provider of Machine Learning Assignment Help services globally and can help you address a wide range of Machine Learning assignment topics. Getting help for Machine learning assignment services to us also makes sense for a whole host of reasons, including -
-
Affordable Pricing Options: Our Machine Learning Assignment Help solutions are priced extremely cost-effectively. With us, you will always obtain the highest return on investment.
-
High-quality Services: Well commented code with proper alignment and make use of multi-level quality checks to ensure that we always provide the highest quality Machine Learning Assignment Help services.
-
Plagiarism Free Solution: The whole solution is written by expert from scratch as following the given instructions in the requirement document.
-
1-on-1 discussion with expert: After solution of the assignment is delivered you can take code walkthrough of the project at very minimum price
-
Tools and Technologies: Our Machine Learning team uses the latest and best tools, editors, and methodologies to provide efficient Machine Learning Assignment solutions.
-
Structured Process: We are a top Machine Learning assignment help service providing company and make use of a streamlined and disciplined process to deliver all our Machine Learning assignment solutions.
-
Experienced Team: Our Machine Learning team is backed by 5 years of solid experience in providing a very wide range of data science assignment help services to a global clientele.
-
Short Turnaround: We will always provide our Machine Learning Assignment Help services on time, every time since we understand how critical time can be for you.
How we Work
Send Requirement
Send your machine learning assignment requirement and tell what you need done in seconds.
Evaluate Project
We'll evaluate your project requirement and assign project to best Expert
Pay safely
Pay using secure payment options like PayPal and by other payment ways
Track Progress
Get Update Everyday and Chat with assigned expert and review their work 24/7.
Pricing Plan
Basic
$ 50 / ₹ 3, 500 Starting
This includes building, training, testing and evaluation of Machine Learning models based on different algorithms like decision trees , SVM etc using pre built packages like Numpy, Scipy, Scikit-learn, Panda, Matplotlib. On data sets which in size are less then 3000 records and are straight forward to implement.
2 Days Delivery
-
1 Variation(Single model training, testing)
-
Include Source Code
Standard
$ 100 / ₹ 6, 500 Starting
3 Days Delivery
-
1 Variation
-
Model Documentation
-
Include Source Code
Advanced
$ 200 / ₹ 14, 500 Starting
5 Days Delivery
-
3 Model Variations
-
Documentation ( 2 - 3 pages)
-
Include Source Code
-
Code Walkthrough
-
Well-documented code.
Our standard services include building different algorithms from scratch without the use of any pre- built machine learning libraries. Also involving analysis and training of different machine learning & deep models on large data sets with great precision.
How the pricing works for Machine Learning Assignment Help
Pricing for machine learning assignment depends upon the time and complexity of the project. Basic Machine Learning tasks, EDA,Training & Testing Model using Sklearn, graphs & plots, Evaluations cost less than advance Machine Learning like implement from scratch, Training on large dataset, hyper parameter, time - series, deep learning, deployment etc. so starting price for 1-on-1 session is 30 USD per hour and you can pay the equivalent amount in any other currency to the country you belongs to. For more details of pricing please check out this pricing page
Machine Learning Assignment Help Forum
Forum for Machine Learning are places where student , learner can post his or her questions , doubts and answer are given by experienced and beginners. Forums are always helpful for learning by doing.Codersarts Machine learning discussion forum are made for student to solve student doubts and questions also we share machine learning projects ideas, Machine Learning examples, Good Machine learning resources, Machine Learning tutorials. For more details you can visit to important Machine Learning forum .
Datasets used in Machine Learning assignment help
When we start working on a Machine learning assignment or project. Selecting the good or right dataset is important and challenging both because success of machine learning models or algorithm is directly proportional. Numerical data, categorical data, time-series data, and text are the most common types of data from a Machine Learning perspective
What factors are to be Considered when Building a Machine Learning Training Dataset? You need to assess and have an answer ready for these basic questions around the data:
-
The number of records to take from the databases.
-
The size of the sample needed to yield expected performance outcomes
-
A "good dataset" does not contains missing values, does not contains aberrant data and Is easy to manipulate (logical structure).
-
A data set consists of roughly two components. The two components are rows and columns. Additionally, a key feature of a data set is that it is organized so that each row contains one observation.
-
The features of a dataset: Each feature, or column, represents a measurable piece of data that can be used for analysis: Name, Age, Sex, Fare, and so on. Features are also sometimes referred to as “variables” or “attributes.” Depending on what you're trying to analyze, the features you include in your dataset can vary widely
-
How to choose a good dataset: The dataset should be rich enough to let you play with it, and see some common phenomena. In other words, it must have at least a few thousand rows (> 3.5 − 4K), and at least 20 − 25 columns. Of course, larger is welcome.
-
The dataset should have a reasonable mix of both continuous and categorical variables
Datasets for Machine Learning - Are you working on Machine Learning project and want to know the best suitable dataset for your project? Codersarts Machine learning engineers, developers and experts have curated the blog post that can help you for selecting project-based datasets that you can use for building your next data science project.
Let's say, we are working on deep learning assignment then which dataset is used in deep leaning. Answer is MNIST dataset, MNIST is one of the most popular deep learning datasets out there. It's a dataset of handwritten digits and contains a training set of 60,000 examples and a test set of 10,000 examples.
Some more dataset based on ml algorithms are given below:
-
Blood Transfusion service center data set - Classification
-
Cervical Cancer Risk factor Dataset - Classification and Clustering
-
Wholesale dataset - Classification and Clustering
-
Online Retail Dataset - Classification, Clustering and Regression
-
Census income dataset - Classification
Are you looking for help in machine learning assignment help in datasets? Connect with Codersarts Machine Learning experts and researcher via 1:1 live session or Email or Chat
Need help in Machine Learning assignment ideas
Are you struggling to find the right machine learning assignment or project ideas? Codersarts is always to help you in project ideation process guidance and ML project proposal writing and also we have listed many predefined project idea to get start working on it.
We have curated the project based on level and use-cases like for beginners, advanced, final year college students and machine learning or data science aspirants.
Also there are lots of project idea are listed over the internet and even some of the project idea are available with source code at the platform like Github but those projects has always compatibility issues, version error, packages used are obsolete and are not in ready to use form. Codersarts Machine learning team resolve such issues and we took an initiatives to make the project code ready to run and fix that code or even implement customer solution from scratch as per user need and demands.Even though you might start working on your own new project ideas. then, how can you make your own machine learning project?
Here are the general steps of developing a machine learning model:
-
Figure out problem to solve in you current organisation or interest
-
Research relevant data and prepared the data over time.
-
Do Features engineering and target variables with basic EDAs
-
Data cleaning and save data in correct file format.
-
Model selection and validations
-
Evaluation matrix and parameter
-
Accepted accuracy
-
Deployment and ready to use for business use cases
There are fundamentals steps not any hard and fast rule you can do as per ease and impact.
Need more Machine Learning project ideas
Whether you are working on Guided project, Capstone Project Or Need Project ideas for solidifying Knowledge and Portfolios Connect with our expert for discuss projects, review code, and learn by doing with mentors.
Machine Learning Assignment Help Samples
Unsupervised Learning - Sample Assignment
The dataset on American College and University Rankings contains information on 1302 American colleges and universities offering an undergraduate program. Our first goal is to estimate these missing values from “similar” records. This will be done by clustering the complete records and then finding the closest cluster for each of the partial records. The missing values will be imputed from the information in that cluster. Read more
Kalman Tracking For Multiple Points - Sample Assignment
In this assignment, you have to implement Kalman tracking for multiple points, simultaneously, through a video. The code will take a video (either as one video file or as individual frames) and output the tracks of the points through the video. Read more
FashionMNIST Using PyTorch - Sample Assignment
In this exercise, you will implement, train and evaluate your neural network using the PyTorch package.First, you will need to install the PyTorch package. Installation instructions were uploaded to the Piazza. Please follow them and discuss issues there. Read more
Big Data Analysis with PySpark - Sample Assignment.
Application of Big Data Analytics on census data can facilitate better decision making in various Government and Industrial sectors such as Healthcare, Education, Finance, Retail, and Housing. One such application is an Income Classifier. In this project, let us take a sample of world census data and build an Income Classifier using various Big Data Techniques described in subsequent sections.
Explore many such machine Learning Sample assignment
Recommended Machine learning Assignment Help Services
Need more Machine Learning help?
-
Learn by doing Machine Learning Projects
From the ML Assignment Help Blog
What’s new and exciting at Codersarts – Machine Learning Assignment Help, Hire Machine Learning Developer, Machine Learning Tutor, blogs and more.
Machine Learning Assignment Help
Looking for an expert to provide you help in machine learning assignment help? or machine learning Homework Help. Codersarts machine learning expert will provide plagiarism free code with with sufficient comments
Machine Learning
Project Help
If you're looking for mini-projects, final year college project, research project, Online job assistance, development project. working on new idea or capstone project in machine learning
Machine Learning Programming Help
Learn to code, debug, Fixing your written code, follow best practices, code reviews, learn conceptual topics of machine learning. Get machine learning course training with theory and code.
Machine Learning Mentorship
Expert guidance. Right when you need it most.
With a direct 1:1 discussion with Machine learning mentor, get help when you’re stuck, and have regular one-on-one calls via Meet, Zoom, Skype
Contact us
Requests are answered in the order they are received instantly.Guaranteed response within 1 business day
Address:
G-69, Sector 63 Noida Pincode. 201301 (INDIA)
Phone:
India: (+91) 7292 00 7989
Landline No: 0120 - 411 8730

bottom of page