Introduction to Explainable AI
- ganesh90
- Jun 17, 2023
- 2 min read
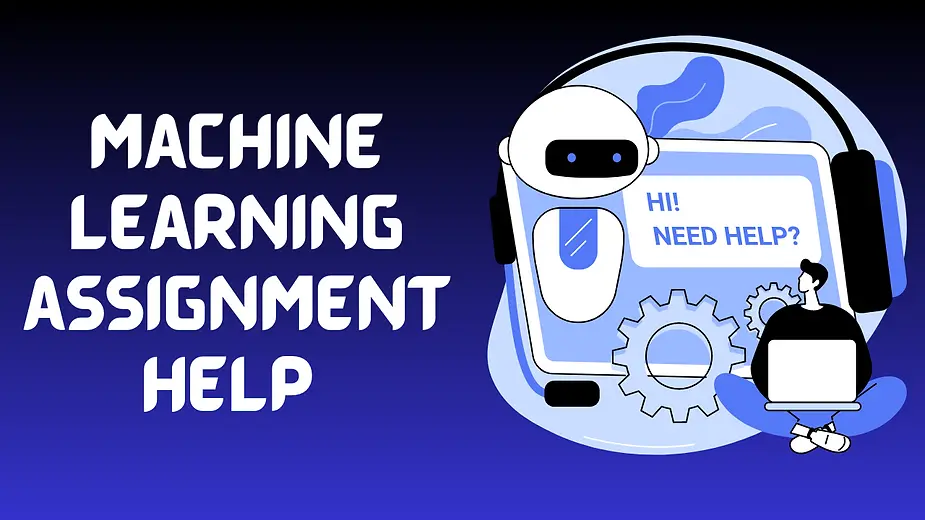
The Importance of Explainable AI
Understanding and interpreting the outcomes of machine learning algorithms can be challenging as AI continues to advance. These algorithms often seem like enigmatic "black boxes" that even their creators cannot fully comprehend or explain. This has led to a growing need for explainable AI (XAI), which comprises processes and techniques that allow humans to grasp and trust the results generated by AI models.
Explainable AI plays a vital role in comprehending the expected impact and potential biases of AI models. It provides insights into model accuracy, fairness, transparency, and decision-making outcomes, enabling organizations to establish trust and confidence when implementing AI in real-world applications.
Understanding the Decision-Making Processes
Explainable AI is essential for organizations to fully comprehend the decision-making processes underlying AI systems. Instead of blindly trusting these systems, organizations can monitor and hold AI models accountable for their actions. Explainable AI helps humans comprehend and explain complex machine learning algorithms, including deep learning and neural networks.
ML models are often considered impenetrable black boxes, with neural networks used in deep learning being particularly difficult for humans to interpret. Bias, which has been a long-standing risk in AI model training, can be rooted in factors such as race, gender, age, or location. Additionally, the performance of AI models can degrade or deviate due to differences between training and production data. Thus, continuous monitoring and management of models are crucial to promote AI explainability while assessing the business impact of these algorithms.
Explainable AI fosters end user trust, facilitates model auditability, and ensures productive utilization of AI. It also mitigates compliance, legal, security, and reputational risks associated with deploying AI in real-world scenarios.
Explainable AI is a crucial requirement for implementing responsible AI, involving the large-scale deployment of AI methods while upholding fairness, model explainability, and accountability. To adopt AI responsibly, organizations need to integrate ethical principles into AI applications and processes, building AI systems based on trust and transparency.
Continuous Evaluation of Models
Explainable AI allows businesses to troubleshoot and enhance model performance while ensuring stakeholders understand the behavior of AI models. Continuous evaluation of models, including monitoring deployment status, fairness, quality, and drift, is essential for scaling AI initiatives. This approach enables businesses to compare model predictions, quantify model risks, and optimize performance.
By displaying positive and negative values in model behaviors and providing data used to generate explanations, model evaluation becomes more efficient.
A data and AI platform can provide feature attributions for model predictions, empowering teams to visually investigate model behavior using interactive charts and exportable documents.
If you need help in machine learning, feel free to contact us.

Comments